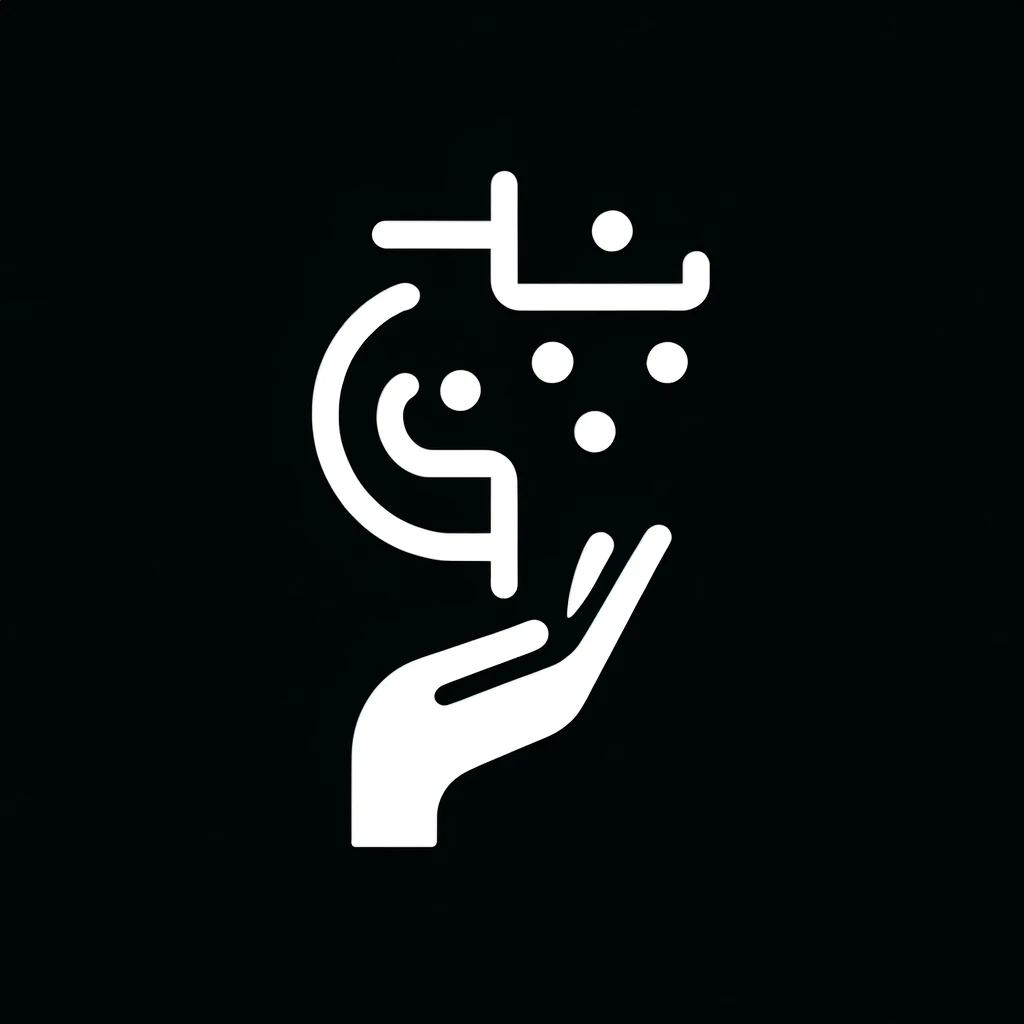
The AI Revolution and Human Adaptation
As we navigate the uncharted waters of the AI revolution, a fundamental question arises: How can human beings maintain their relevance in a world increasingly dominated by intelligent machines? The answer lies in understanding and cultivating ‘low-compressibility’ skills—those human capabilities that are not easily replicated or replaced by AI.
Understanding Low-Compressibility Skills
In the realm of data, ‘compressibility’ refers to the extent to which information can be condensed without loss. Applying this concept to human skills, low-compressibility skills are those complex, nuanced, and inherently human traits that resist simplification and automation.
The Creative Edge: Beyond AI’s Reach
Creativity, the hallmark of human ingenuity, stands at the forefront of low-compressibility skills. It’s not just about artistic endeavors but extends to innovative thinking, problem-solving, and the ability to conceive ideas that are truly ‘outside the box’. While AI can generate art or music based on existing patterns, it lacks the capacity for original thought, the spontaneity of inspiration, and the depth of emotional connection that human creativity embodies.
- Art and Design: In the world of art and design, creativity manifests in the ability to convey complex emotions, societal critiques, and personal narratives. These realms require an understanding of human experiences and cultural contexts that AI cannot grasp.
- Business Innovation: In the business world, creativity is about envisioning novel solutions to complex problems, identifying unmet market needs, and pioneering new business models. It involves a deep understanding of human behavior, market dynamics, and the ability to take calculated risks—areas where AI tools serve as aids but cannot lead.
Emotional Intelligence: The Human Connection
Emotional intelligence (EQ) is a distinctly human attribute that AI has yet to mimic successfully. EQ involves understanding and managing one’s own emotions and empathetically navigating others’ emotions. This skill is crucial in professions that rely on interpersonal relationships, emotional support, conflict resolution, and leadership.
- Healthcare and Therapy: In healthcare, the ability to provide empathetic care and understand patient needs goes beyond clinical diagnoses. Therapists, counselors, and caregivers rely heavily on EQ to connect with and support their clients.
- Leadership and Management: Effective leadership is deeply rooted in EQ. It involves motivating teams, understanding individual team members’ strengths and weaknesses, and fostering a positive and productive work environment. These are nuanced tasks that AI cannot replicate.
Complex Problem Solving and Critical Thinking
While AI excels at processing data, human beings bring context, ethical considerations, and creative problem-solving to the table. Complex problem-solving involves understanding multifaceted issues, considering diverse perspectives, and devising solutions that balance various factors.
- Policy-making and Strategy: In the spheres of policy-making and strategic planning, complex problem-solving is essential. It requires a deep understanding of societal issues, economic trends, and the potential long-term impacts of decisions.
- Scientific Research: In scientific research, complex problem-solving combines empirical data analysis with theoretical understanding, creativity, and the ability to hypothesize and innovate. This blend of skills is something AI can assist with but cannot fully undertake.
Adaptability and Lifelong Learning: The Human Superpower
The ability to adapt and learn continuously is perhaps the most crucial skill in the AI era. As technology evolves, so must our skills and knowledge. This adaptability goes beyond merely acquiring new technical skills; it involves a mindset of lifelong learning, openness to change, and the capacity to apply knowledge in ever-changing contexts.
- Technological Adaptability: Keeping pace with technological advancements is essential. This doesn’t mean competing with AI but rather understanding how to leverage it effectively in various domains.
- Cross-disciplinary Learning: The future belongs to those who can combine knowledge from multiple fields—technology, humanities, arts, and sciences—to create innovative solutions to complex problems.
Compressible Skills
- Data Entry: Manual input of data into computer systems.
- Basic Arithmetic Calculations: Simple mathematical operations.
- Routine Clerical Work: Standard office tasks like filing and organizing.
- Basic Customer Service Responses: Standardized customer interactions.
- Assembly Line Work: Repetitive manufacturing tasks.
- Basic Bookkeeping: Standard financial record-keeping.
- Simple Coding Tasks: Basic programming following set patterns.
- Scripted Sales Calls: Standardized sales pitches and interactions.
- Translation of Common Phrases: Basic language translation without nuance.
- Form Filling: Completing standard forms or applications.
- Ticket Booking and Reservation Services: Routine booking tasks.
- Simple Quality Control Checks: Basic product inspection.
- Standardized Testing and Grading: Grading work based on clear criteria.
- Routine Cleaning Services: Standard cleaning tasks in predefined environments.
- Cataloging and Indexing: Systematic ordering of information.
- Basic Content Moderation: Filtering content based on clear guidelines.
- Template-Based Writing: Creating content based on set templates.
- Simple Technical Support: Basic troubleshooting following a script.
- Inventory Management: Basic tracking and recording of stock.
- Transcription of Clear Audio: Converting spoken words to text.
Uncompressible Skills
- Creative Problem Solving: Developing novel solutions to complex issues.
- Strategic Planning: Long-term planning with innovative thinking.
- Empathy and Emotional Support: Understanding and addressing emotional needs.
- Advanced Negotiation: Handling complex and nuanced negotiations.
- Original Artistic Creation: Producing unique art, music, or literature.
- Innovative Scientific Research: Pioneering new scientific discoveries.
- Complex Project Management: Overseeing intricate and multifaceted projects.
- Ethical Decision Making: Navigating moral dilemmas.
- Advanced Medical Diagnosis and Treatment: Handling complex medical cases.
- Inspiring Leadership: Motivating and guiding diverse teams.
- Critical Thinking: Analyzing and evaluating complex information.
- Counseling and Therapy: Providing in-depth psychological support.
- Advanced Legal Analysis and Argumentation: Dealing with intricate legal issues.
- Cross-Cultural Communication and Understanding: Navigating and understanding diverse cultural contexts.
- Crisis Management and Response: Handling emergency situations effectively.
- Entrepreneurial Initiative: Launching and managing new business ventures.
- In-depth Journalism and Investigative Reporting: Deep, insightful reporting on complex topics.
- Human-Centered Design and UX: Designing with a focus on human experience.
- Advanced Software Development: Creating complex and innovative software solutions.
- Personalized Education and Coaching: Tailoring education to individual needs and goals.
Ethical Considerations and Philosophical Insights
In an era where technology intersects increasingly with ethical dilemmas, the human capacity for moral reasoning remains crucial. AI lacks the ability to engage in ethical debates or make decisions that consider societal values, cultural nuances, and moral implications.
- Ethical Leadership: In business and technology, ethical leaders are needed to navigate the moral implications of AI and other emerging technologies.
- Philosophical and Cultural Understanding: Understanding the philosophical underpinnings of our actions and the cultural contexts in which technology operates is vital. This understanding shapes how technology is developed, deployed, and regulated.
Embracing Our Human Qualities in the AI Era
The advent of AI is not a signal of human obsolescence but an opportunity to reaffirm and reinvigorate the uniquely human skills that define us. By focusing on low-compressibility skills, we can ensure our relevance and thrive in the AI-driven future. In this symphony of progress, AI may provide the rhythm, but human creativity, empathy, and ingenuity are the melody that will lead us forward.