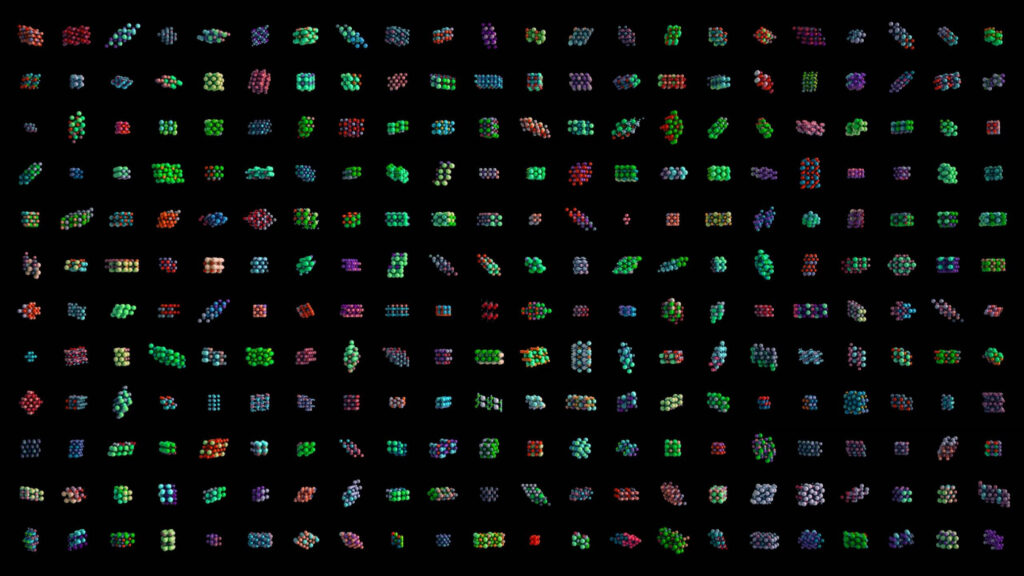
Materials innovation is central to technological progress, from powering modern devices with lithium-ion batteries to enabling efficient solar panels and carbon capture technologies. Yet, discovering new materials for these applications is an arduous process, historically reliant on trial-and-error experiments or computational screenings. Microsoft’s MatterGen is poised to change this paradigm, leveraging cutting-edge generative AI to revolutionize material discovery.
The Challenge in Material Design
Traditionally, researchers sift through vast databases of known materials or rely on high-throughput experiments to identify candidates with specific properties. While computational approaches have sped up this process, they are still limited by the need to evaluate millions of candidates from existing data. This bottleneck often misses novel and unexplored possibilities. MatterGen offers a transformative approach, generating novel materials directly based on user-defined properties like chemical composition, mechanical strength, or electronic and magnetic characteristics.
What Is MatterGen?
MatterGen is a diffusion-based generative model designed to create stable, unique, and novel (S.U.N.) inorganic materials. Unlike traditional material screening, which filters pre-existing datasets, MatterGen uses advanced AI algorithms to construct entirely new materials from scratch.
This model employs 3D diffusion processes, iteratively refining atom positions, lattice parameters, and chemical compositions to meet desired property constraints. Its architecture accommodates material-specific complexities like periodicity and crystallographic symmetries, ensuring both stability and functionality.
Key Innovations in MatterGen’s Architecture
- Diffusion Process Tailored for Materials: MatterGen’s architecture uses a novel forward and reverse diffusion approach to refine atomic structures from noisy initial configurations, ensuring equilibrium stability.
- Fine-Grained Control Over Design Constraints: The model can be conditioned to generate materials with specific space groups, chemical systems, or properties like high magnetic density or bulk modulus.
- Scalable Training Data: Leveraging over 600,000 entries from the Alexandria and Materials Project databases, MatterGen achieves superior performance compared to existing methods like CDVAE and DiffCSP.
- Novelty Through Disordered Structure Matching: A sophisticated algorithm evaluates whether generated materials represent genuinely new compositions or ordered variants of known structures.
Validation Through Experimentation
MatterGen’s capabilities extend beyond theoretical predictions. Collaborating with experimental labs, researchers synthesized TaCr₂O₆, a novel material generated by the model to meet a target bulk modulus of 200 GPa. Despite minor cationic disorder in the crystal structure, the material closely matched its computational design, achieving an experimentally measured bulk modulus of 158 GPa. This milestone demonstrates MatterGen’s practical applicability in guiding real-world material synthesis.
Comparative Performance
MatterGen significantly outperforms its predecessors:
- Higher Stability Rates: The generated structures align closer to DFT (Density Functional Theory)-computed energy minima, with an average RMSD (Root Mean Square Deviation) 15 times lower than competing models.
- Unprecedented Novelty: Leveraging its advanced dataset and refined diffusion processes, MatterGen generates a higher proportion of novel materials than previous approaches like CDVAE.
- Property-Specific Design: The model excels in constrained design scenarios, such as creating materials with high bulk modulus or low supply-chain risk.
Broader Implications
The success of MatterGen heralds a new era in material science, shifting the focus from searching databases to generative design. By integrating MatterGen with complementary tools like MatterSim—Microsoft’s AI emulator for material property simulations—researchers can iteratively refine designs and simulations, accelerating the entire discovery process.
Applications Across Industries
- Energy Storage: Novel materials for high-performance batteries and fuel cells.
- Carbon Capture: Adsorbents optimized for CO₂ sequestration.
- Electronics: High-efficiency semiconductors and magnets for next-gen devices.
Open Access for the Research Community
True to Microsoft’s commitment to advancing science, the MatterGen code and associated datasets are available under an open MIT license. Researchers can fine-tune the model for their specific applications, fostering collaborative advancements in materials design.
The Road Ahead
MatterGen represents just the beginning of generative AI’s potential in material science. Future work will aim to address remaining challenges, including synthesizability, scalability, and real-world integration into industrial applications. With continued refinement, generative AI promises to unlock innovations across fields, from renewable energy to advanced manufacturing.