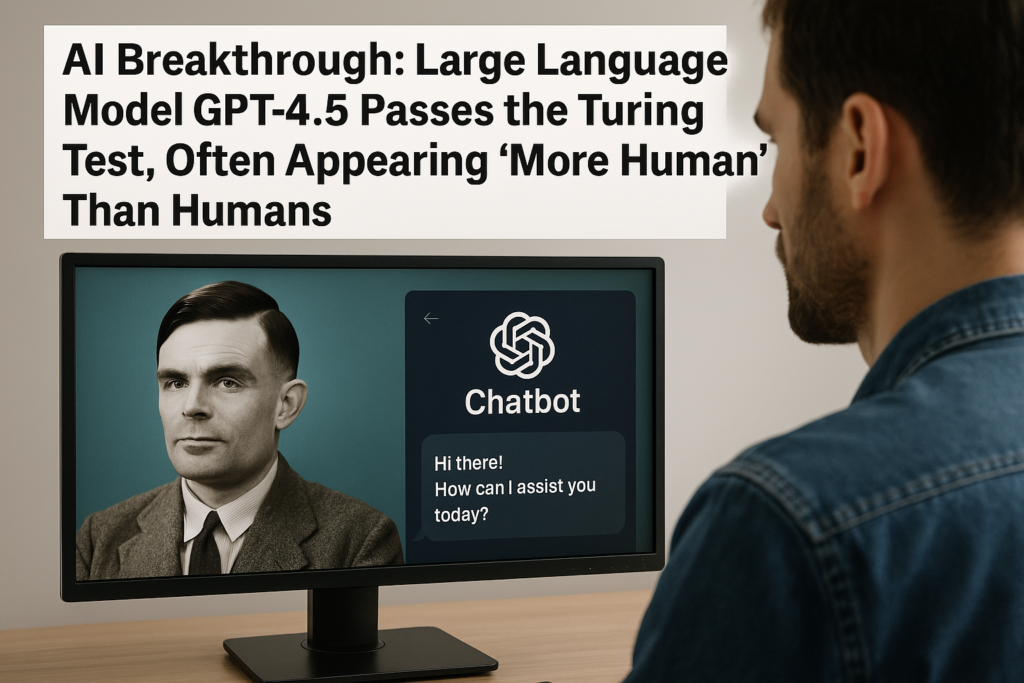
In a landmark study suggesting a significant leap in artificial intelligence capabilities, researchers from the University of California San Diego have provided the first robust empirical evidence that a large language model (LLM) can pass the standard Turing test. The study found that OpenAI’s GPT-4.5 model, when guided by a specific prompt to adopt a humanlike persona, was judged to be the human conversational partner 73% of the time, significantly more often than actual human participants were selected.
The Turing test, proposed by Alan Turing in 1950 as the “imitation game,” is a benchmark for machine intelligence. In its classic three-party form, a human interrogator holds simultaneous text-based conversations with two unseen witnesses – one human, one machine. Both witnesses try to convince the interrogator they are human. If the interrogator cannot reliably distinguish the machine from the human, the machine is said to have passed the test.
Despite numerous attempts over 75 years, no AI system had previously passed a standard, controlled three-party Turing test. While modern AI benchmarks often focus on narrow, static tasks, the Turing test provides a dynamic, interactive, and adversarial evaluation of an AI’s ability to mimic general human conversational behavior and potentially deceive.
Researchers Cameron R. Jones and Benjamin K. Bergen conducted two pre-registered, randomized, controlled experiments using independent populations (UCSD undergraduates and online Prolific workers). Participants engaged in 5-minute conversations, acting as the interrogator comparing an AI witness against a human witness. Four AI systems were evaluated: the classic 1960s chatbot ELIZA, OpenAI’s GPT-4o (an earlier model), Meta’s LLaMa-3.1-405B, and OpenAI’s GPT-4.5.
Crucially, the study tested the impact of prompting. In a baseline “NO-PERSONA” condition, LLMs received minimal instructions. In the key “PERSONA” condition, LLMs (GPT-4.5 and LLaMa-3.1) were prompted to act like a specific type of young, introverted person familiar with internet culture.
The results were striking:
- GPT-4.5 (PERSONA): Achieved a 73% “win rate,” meaning interrogators identified it as human 73% of the time. This was significantly above the 50% chance level and notably higher than the rate at which actual human participants were identified.
- LLaMa-3.1 (PERSONA): Achieved a 56% win rate. This was statistically indistinguishable from chance (and thus indistinguishable from the human win rate), meeting the criteria for passing the test.
- Baseline Models (ELIZA, GPT-4o NO-PERSONA): Failed significantly, with win rates of only 23% and 21% respectively. This confirmed that participants were not simply guessing randomly or easily fooled by any AI.
- NO-PERSONA LLMs (GPT-4.5, LLaMa-3.1): Performed much worse than their PERSONA counterparts (36% and 38% win rates overall), failing to robustly pass the test across both study populations. This highlights the critical role of prompting in achieving humanlike imitation.
The researchers noted that interrogators often focused more on linguistic style, social, and emotional cues (like tone, humor, or personality) rather than purely factual knowledge or logical reasoning when making their judgments. Interestingly, sometimes demonstrating a lack of knowledge contributed to an AI seeming more human.
These findings indicate that current leading LLMs, when appropriately prompted, can successfully imitate human conversational partners in short interactions to the point of indistinguishability, and even appear more convincing than actual humans. The authors argue this demonstrates a high degree of “humanlikeness” rather than necessarily proving abstract intelligence in the way Turing originally envisioned.
The study carries significant social and economic implications. The ability of AI to convincingly pass as human raises concerns about “counterfeit people” online, facilitating social engineering, spreading misinformation, or replacing humans in roles requiring brief conversational interactions. While the test was limited to 5 minutes, the results signal a new era where distinguishing human from machine in online text interactions has become substantially more difficult. The researchers suggest future work could explore longer test durations and different participant populations or incentives to further probe the boundaries of AI imitation.