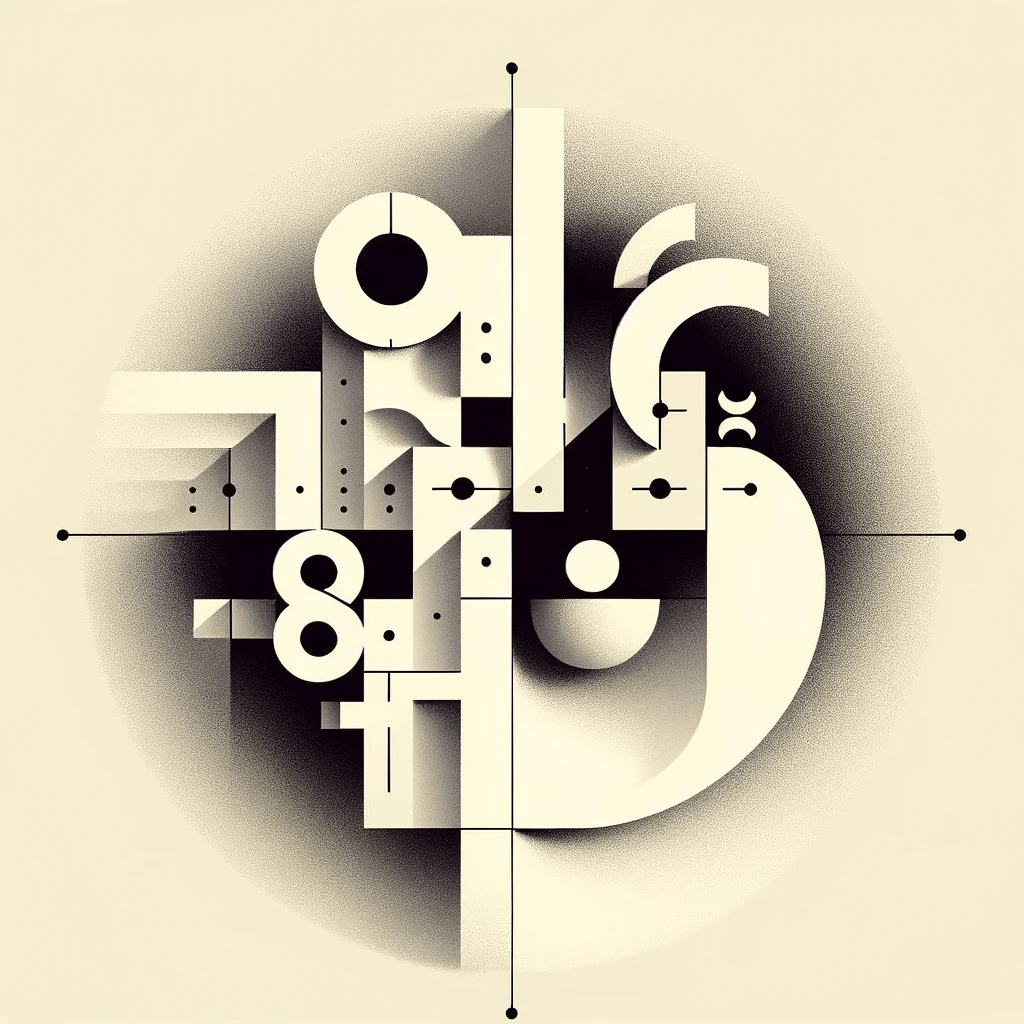
DeepMind’s latest innovation, FunSearch, marks a significant leap in the realm of mathematical sciences through the application of Large Language Models (LLMs). Published on December 14, 2023, by Alhussein Fawzi and Bernardino Romera Paredes, this groundbreaking technology represents a paradigm shift in how we approach and solve complex mathematical and computational problems.
Breaking New Ground with LLMs
LLMs, known for their ability to read, write, and code, traditionally assisted in problem-solving by combining various concepts. FunSearch, however, takes this a step further by not only assisting in problem-solving but also making novel discoveries in mathematical sciences. This is particularly noteworthy because LLMs, despite their capabilities, have been prone to producing factually incorrect information, commonly referred to as “hallucinations”. FunSearch counters this by pairing a pre-trained LLM with an automated evaluator to filter out these inaccuracies, allowing the system to evolve initial ideas into verifiable new knowledge.
Innovative Approach: Evolutionary Method and Practical Applications
The core of FunSearch’s methodology is an evolutionary process powered by LLMs. It starts with a user-defined problem described in code, initiating a cycle where the LLM generates new program ideas, which are then automatically evaluated and refined. This iterative process results in a self-improving loop, enhancing the quality of solutions over time. Remarkably, FunSearch has been instrumental in finding new solutions to the cap set problem – a long-standing challenge in mathematics – and improving algorithms for the bin-packing problem, demonstrating its practical utility in diverse applications.
Beyond Traditional Computing: The Advantages of FunSearch
What sets FunSearch apart from conventional computing methods is its ability to generate programs that elucidate the process of arriving at solutions, rather than just the solutions themselves. This characteristic aligns closely with the scientific method of explaining phenomena and discoveries. Moreover, FunSearch prefers compact programs, reducing complexity and enhancing the interpretability of its outputs. This feature not only aids in understanding but also in refining and improving solutions through collaborative human-machine interactions.
Addressing Real-World Challenges
FunSearch’s versatility extends beyond theoretical problems to practical challenges like the bin-packing problem, crucial in various industrial applications. Its ability to generate tailored programs that outperform existing heuristics highlights its potential in optimizing real-world systems efficiently. Unlike other AI approaches that might require significant resources, the solutions provided by FunSearch are easily deployable, offering immediate practical benefits.
Looking Forward: The Future of LLM-Driven Discovery
As LLMs continue to evolve, so will FunSearch. Its current success is just the beginning, with plans to expand its capabilities to tackle a broader spectrum of scientific and engineering challenges. This advancement positions FunSearch and similar LLM-driven technologies as future mainstays in solving complex problems in science and industry.
Leave a Reply